Welcome to imbalanced-ensemble documentation!
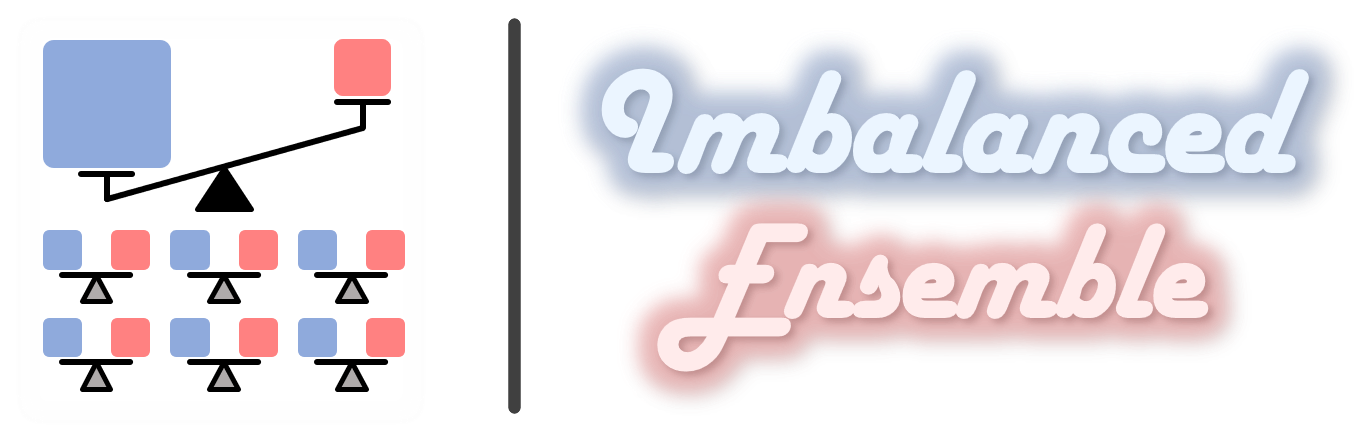
[Github] [Gallery] [PyPI] [Changelog] [Source] [Download] [知乎/Zhihu] [中文README] [arXiv]
Date: Jul 22, 2023 Version: 0.2.1
Paper: IMBENS: Ensemble Class-imbalanced Learning in Python
Citing US
If you find IMBENS helpful in your work or research, we would greatly appreciate citations to the following paper [PDF]:
@article{liu2023imbens,
title={IMBENS: Ensemble Class-imbalanced Learning in Python},
author={Liu, Zhining and Kang, Jian and Tong, Hanghang and Chang, Yi},
journal={arXiv preprint arXiv:2111.12776},
year={2023}
}
imbalanced-ensemble (IMBENS, imported as imbens
) is a Python toolbox
for quick implementation, modification, evaluation, and visualization of ensemble learning
algorithms for class-imbalanced data.
It was built on the basis of scikit-learn
and imbalanced-learn.
IMBENS includes more than 15 ensemble imbalanced learning (EIL) algorithms, from the
classical SMOTEBoost (2003) and RUSBoost (2010) to recent SPE (2020), from resampling-based
methods to cost-sensitive ensemble learning.
IMBENS is featured for:
Unified, easy-to-use APIs, detailed documentation and examples.
Capable for out-of-the-box multi-class imbalanced (long-tailed) learning.
Optimized performance with parallelization when possible using joblib.
Powerful, customizable, interactive training logging and visualizer.
Full compatibility with other popular packages like scikit-learn and imbalanced-learn.
API Demo:
>>> from imbens.ensemble import SelfPacedEnsembleClassifier
>>> from imbens.datasets import generate_imbalance_data
>>> from imbens.utils import evaluate_print
>>> from imbens.visualizer import ImbalancedEnsembleVisualizer
>>>
>>> X_train, X_test, y_train, y_test = generate_imbalance_data(
... n_samples=200, weights=[.9,.1], test_size=.5)
>>>
>>> clf = SelfPacedEnsembleClassifier() # initialize ensemble
>>> clf.fit(X_train, y_train)
>>>
>>> y_test_pred = clf.predict(X_test) # predict labels
>>> y_test_proba = clf.predict_proba(X_test) # predict probabilities
>>>
>>> evaluate_print(y_test, y_test_pred, "SPE") # performance evaluation
SPE balanced Acc: 0.972 | macro Fscore: 0.886 | macro Gmean: 0.972
>>>
>>> visualizer = ImbalancedEnsembleVisualizer() # initialize visualizer
>>> visualizer.fit({'SPE': clf})
>>>
>>> visualizer.performance_lineplot() # performance visualization
>>> visualizer.confusion_matrix_heatmap() # prediction visualization
Getting Started
API